Why can’t humanoid robots bear the weight of intelligence?
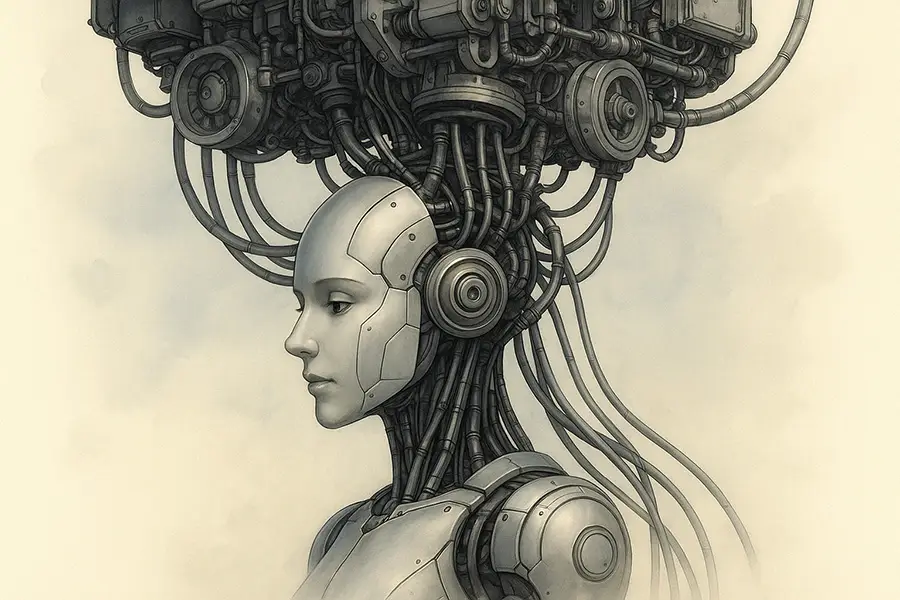
Humanoid robots are a key embodiment of intelligence, yet the integration of complex AI models into these platforms still has a long way to go
By Lead Leo Research Institute
As generative AI rapidly evolves, humanoid robots are seen as the ideal vessel for embodied intelligence. However, deploying AI models into these complex platforms is proving far more difficult than expected. According to Rao Lijie, a comprehensive industry analyst at LeadLeo Research Institute, despite growing policy support and capital investment, the integration of large models into humanoid robots could face significant technological and commercial challenges.
Hardware limitations are the most immediate obstacle. For example, models like GPT-4, with hundreds of billions of parameters, require tens of gigabytes of memory and over 100 trillion operations per second (TOPS) of computing power. By comparison, most current humanoid robots use embedded computing platforms — such as Nvidia’s Jetson series — that offer only 10 to 50 TOPS, which is far from sufficient. As a result, these robots often rely on cloud-based processing, which consumes 5W to 10W of power per hour via 5G or 6G modules and introduces latency and potential disconnection risks — undermining real-time decision-making.
Alternately, running large models locally requires high-end AI processors such as Nvidia’s Orin, which typically consumes 15W to 30W of power. Given that most humanoid robots have battery capacities between 1 kWh to 3 kWh, this would significantly reduce operating time — by as much as 75%. For robots designed for long-duration autonomous operation, this becomes a fatal shortcoming.
Large gap in control response
Even if computing power were sufficient, synchronizing sensor input with model inference remains a major challenge. Humanoid robots must complete the perception-understanding-action loop within 100 milliseconds to 300 milliseconds. But inference delays in large models often extend to several seconds — falling short of the requirements for real-time control.
For instance, when a robot receives voice or visual input, it must immediately decide whether and how to act. But excessive inference delay can cause a lag or errors in execution — posing serious risks in complex or high-stakes environments.
Beyond the technical challenges, commercialization remains an unresolved core issue. High computational demands translate into high hardware costs. Equipping a robot with a GPU such as Nvidia’s A100 — priced around $15,000 — can easily push the total system cost into the tens of thousands of dollars, far exceeding the sub-$10,000 price point generally considered acceptable in the consumer market. This directly squeezes the commercial return on investment.
In deployment scenarios, humanoid robots running large models are mostly limited to semi-closed environments like warehouses or factories. Adapting to open environments — such as home care or retail assistance — remains difficult due to insufficient model generalization across varied spaces, semantics and behavioral demands. This lack of adaptability weakens the foundation for stable commercial growth.
For humanoid robots to truly harness the power of AI models, simultaneous breakthroughs are needed in computational power, power management, response latency, and scenario generalization. In the short term, smaller or mid-sized domain-specific models may offer more viable paths for applications in industrial inspection, medical assistance and other fields. In the long run, as lightweight AI chips and surrounding computing architectures continue to mature, large AI models may finally become the true “brains” of humanoid robots — ushering embodied intelligence into broader commercial frontiers.
LeadLeo Research Institute is an original content platform for research on banks and companies and an innovative digital research service provider with nearly 100 senior analysts. You can contact the platform at CS@leadleo.com.
This article was originally published on The Time Weekly by Zhu Chengcheng.
This commentary is the views of the writer and does not necessarily reflect the views of Bamboo Works
To subscribe to Bamboo Works weekly free newsletter, click here